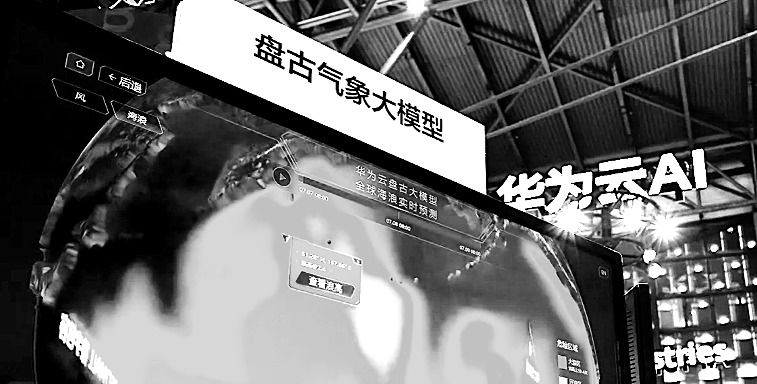
Beyond the challenges of safety and security posed by artificial intelligence, what environmental issues does its development raise? For Hamilton Mann, safe and trustworthy AI cannot be detached from a sustainable dimension. In this paper, the author provides an overview of the environmental issues associated with the development of AI while outlining potential regulatory pathways.
The race for artificial intelligence is on, and we cannot afford to overlook its systemic impact on Earth's resources.
It's undeniable that one of the most significant applications of AI lies in its potential to play a pivotal role in the accelerated fight against climate change, largely due to its ability to analyze and interpret large quantities of real-time data. For example, using machine-learning algorithms, energy management systems can learn consumption habits and automatically adjust energy use to minimize waste while helping to forecast electricity demand.
Similarly, AI can enhance environmental monitoring by analyzing satellite images to detect extreme weather events, changes in ecosystems like deforestation or ice melt, and trigger early warnings for proactive intervention. In agriculture, smart algorithms can optimize the use of water and fertilizers, thereby reducing greenhouse gas emissions.
However, while AI is one of the most promising solutions for ecological transition and combating climate change, it also poses a serious environmental threat.
The mightier the AI, the more energy it consumes.
In an increasingly AI-inhabited world, as we marvel at the astounding abilities of AI, it's crucial to consider the power-hungry infrastructure that sustains and propels it.
The crux of the issue lies in the massive computational power required for its smooth operation. This technology, particularly 'Deep Learning,' involves intensive mathematical operations that consume substantial amounts of energy.
Training these models on vast datasets often involves running the algorithm repeatedly on the same set of data—fine-tuning parameters for accuracy and reliability—thereby escalating energy consumption.
Hardware components, specialized computational accelerators like GPUs (Graphic Processing Units), NPUs (Neural Processing Units), and ASICs (Application-Specific Integrated Circuits), are also energy-intensive, despite improvements in energy efficiency.
Moreover, large-scale AI operations are often conducted in data centers, server farms that themselves consume colossal amounts of electricity, rivaling even the consumption levels of entire cities.
A study from the University of Massachusetts revealed that training a substantial AI model for natural language processing could result in emissions of nearly 300,000 kilograms of carbon dioxide equivalent. This is roughly five times the lifetime emissions of an average car in the United States, including its manufacturing.
Indeed, the large data farms and high-performance computing infrastructures indispensable to AI training and inference still heavily rely on fossil fuels like coal, natural gas, and oil for the electricity they consume.
The United States and China host some of the largest data centers. Singapore is also becoming a major hub for these installations. Canada, along with Northern European countries like Sweden, Finland, and Norway, are attractive locations for these centers due to their cold climates and energy sources. These centers are also expanding in India. Finally, France is also a significant host for these centers, benefiting from low carbon-emitting energy, thanks to nuclear power.
As AI continues to evolve and data becomes the new 'black gold,' the energy consumption required for its development constitutes an urgent concern.
AI: a major water consumer.
The swift proliferation of AI applications and the expansion of data-driven technologies also present challenges concerning water use.
The insatiable thirst for power in AI computations produces substantial amounts of heat in data centers, necessitating efficient cooling systems to maintain optimal performance.
Many data centers utilize water-based cooling methods, thus impacting the overall water consumption of a country or region.
For data centers located in areas already grappling with water scarcity, competition for water resources intensifies, rightfully raising concerns.
Indeed, some regions of the United States, Northern China, and Northern Europe are experiencing water shortages due to drought periods and high demand. Singapore, with its limited water resources, heavily relies on imports and desalination. Countries in the Asia-Pacific region, especially those with arid climates, are also facing water scarcity. India's situation is similar, made even more pressing by the country's population growth. Canada is also not exempt from these issues. In France, the increasing frequency of dry and warm climates, along with early heatwaves, impacts agricultural activities and water availability in certain areas.
The significant water consumption in data centers can thus put local ecosystems under severe strain.
Furthermore, excessive water withdrawals, especially when sourced from rivers, lakes, or underground aquifers, can harm aquatic life, disrupt natural habitats, and lower water levels, affecting local biodiversity and ecosystems.
Climate change further exacerbates water stress in different regions: extreme weather events such as droughts or floods can disrupt water supply.
The United Nations anticipates that by 2030, population growth combined with climate change will result in an unprecedented water crisis, with global demand for freshwater expected to exceed supply by 40%.
Simultaneously, researchers from the Massachusetts Institute of Technology project that by 2050, 5 billion (52%) of the estimated 9.7 billion people on Earth will live in areas under water stress.
In a context where the AI landscape intensifies competition for water, conflicts with other essential uses, such as agriculture, industry, and everyday necessities, might arise, potentially impacting its availability and quality.
AI: a contributor to electronic waste.
The rapid pace of hardware component development essential for AI results in frequent upgrades and replacements of GPUs, ASICs, and other specialized chips.
This advancement contributes to the creation of electronic waste and the depletion of essential minerals like coltan, cobalt, and other scarce natural resources used in manufacturing these electronic components.
In 2022, the world produced 59.4 million metric tons of electronic waste, with recycling rates not exceeding 20% in the most optimistic estimates.
The remainder, which contains hazardous materials such as lead, mercury, and cadmium, ends up in landfills or is informally recycled, thereby contributing to electronic waste pollution that poses environmental and health risks.
The inadequate management of electronic waste has become an urgent, large-scale issue, affecting various countries worldwide, including China, India, Nigeria, Ghana, Pakistan, Bangladesh, Vietnam, the Philippines, Egypt, and Mexico.
Several factors position these nations at the forefront of this challenge, as they've become prime destinations for electronic waste imports. The allure of low-cost recycling solutions makes them popular choices for processing this waste, leading to a significant accumulation of these modern gadgets eventually found in the environment.
The lack of a well-developed recycling industry combined with the absence of strict regulations hinders their ability to cope with this influx of waste. This creates an environment conducive to the development of improper and dangerous recycling practices.
Amplified by the rush towards AI, the ecological consequences of inadequate electronic waste management further intensify environmental challenges. This affects the quality of air, soil, and water, leading to severe pollution and significant health risks.
The development of trustworthy AI must inherently be sustainable.
While human oversight, transparency, explainability, technical robustness, security, privacy, data governance, non-discrimination, and fairness are certainly necessary characteristics for trustworthy AI, they are still insufficient when it comes to the climate challenge.
It is imperative to consider the environmental impact of AI, whether it's in terms of energy consumption, water use, or the generation of electronic waste that it induces.
There is still time to change course so as not to jeopardize the health of our already vulnerable planet.
From the perspective of development practices, here are some avenues that could be explored:
- AI infrastructure should transition to a primary reliance on renewable energy sources, along with eco-friendly cooling mechanisms.
- Dynamic resource allocation algorithms, intelligently distributing computational tasks based on real-time demand and available resources, should be implemented.
- New techniques for training AI models with lower energy consumption must be explored.
- Algorithms for AI inference (the process of evaluating the effectiveness of a trained AI model, through which the model makes predictions or decisions based on data not used during its training) capable of dynamically adjusting computational complexity based on energy constraints should be developed.
- Investments in data quality enhancement techniques should be made, thereby reducing the need for data storage.
- Manufacturers of AI components should adopt circular economy principles, prioritizing recycling and responsible disposal.
From a regulatory perspective, the following could be developed and implemented:
- Ethical guidelines concerning data collection and usage, aimed at avoiding unnecessary data storage.
- Guidelines for environmentally friendly data labeling practices.
- A standardized "green certification" program for AI to encourage environmentally responsible practices.
- Regulations requiring environmental impact assessments for large-scale AI projects.
- Extended liability for AI component manufacturers, making them responsible for the recycling of their products.
- Carbon pricing mechanisms that assign a price to carbon emissions generated during the development and training of AI.
From a research and development standpoint:
- AI research should focus on the development of energy-efficient algorithms that do not compromise performance.
- The development and adoption of open-source AI frameworks that integrate sustainability principles and environmentally-friendly algorithms should be encouraged.
- Research and development on AI model architectures should prioritize resource utilization efficiency.
- Research on decentralized AI systems that distribute computing tasks among devices such as smartphones, edge devices, and IoT nodes should be accelerated.
- Emphasis should be placed on researching techniques for designing eco-friendly computational circuits for AI, such as neuromorphic computing, which saves energy by mimicking the brain.
Developing trustworthy AI requires responsible commitment.
This is an indispensable condition to build a future in which we can all trust.
This article was published in french and in english on Institut Montaigne
Comments